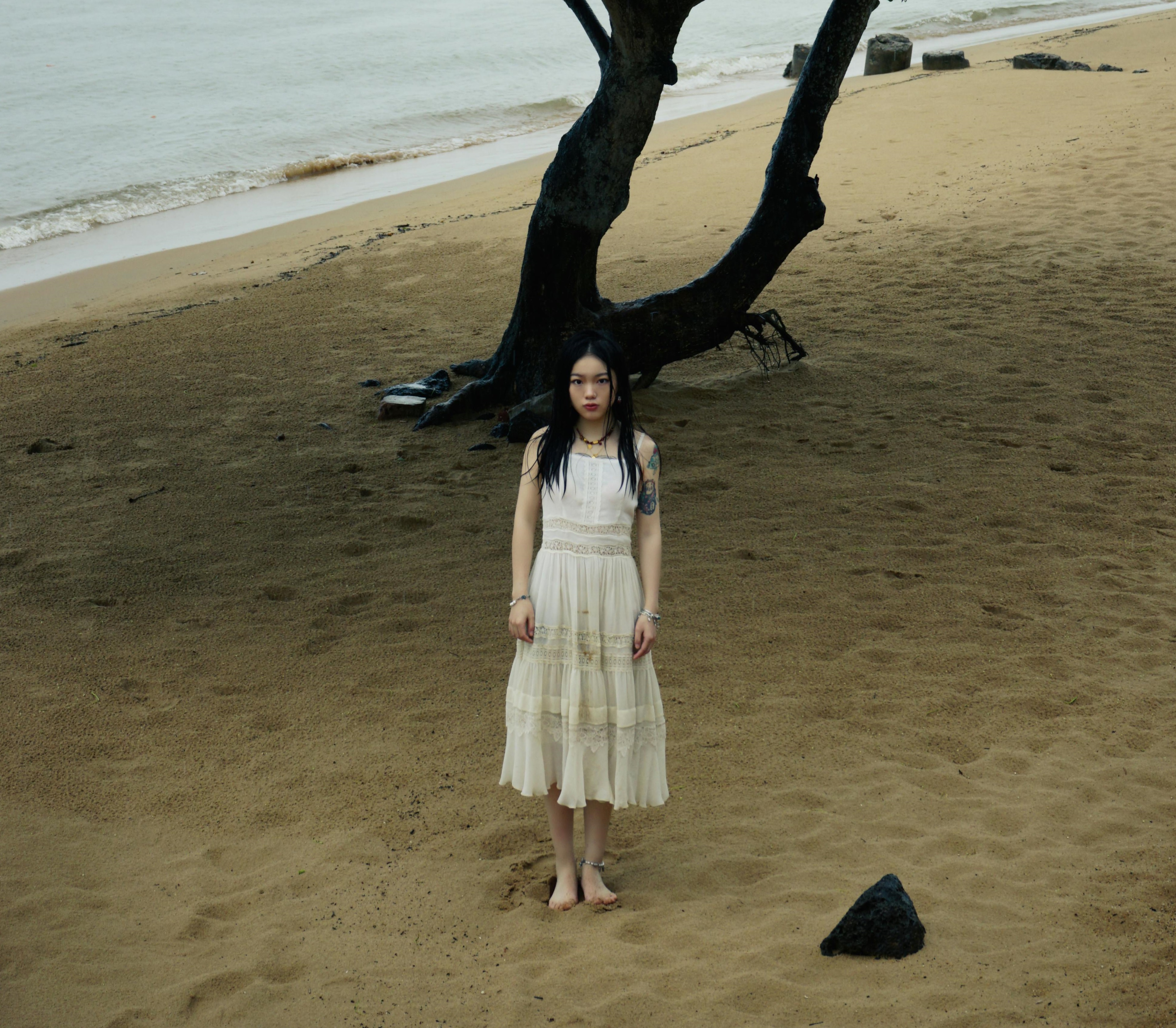
To past Eli - here is what you need to do
7/11/2024
Eli Gacasan
1. Prioritize practice over theory (if possible, learn theory along the way)
The modern age has ushered in a proliferation of information and data. Whether neatly structured in an Excel file, stored in Amazon’s data centers, embedded in the health records of EU countries digitizing medical prescriptions, or filed away in university HR departments, data is everywhere.
About four years ago, I entered university with high hopes of mastering everything there is to know about data and the tools used to analyze it. My naivety, however, laid the foundation for a more conservative understanding of statistics.
In statistical hypothesis testing, a conservative test is less likely to reject the null hypothesis, making it less prone to identifying significant effects-or, more simply, interesting results.
Statistical hypothesis testing is just one part of the trio that constitutes statistical inference, alongside point estimation and confidence intervals. This trio has defined classical statistics for the past century. As I delved deeper into the field, I was gradually disillusioned to find that life as a data analyst was less about the grand theories and more about the practicalities—datasets, both old and new models, and the intriguing questions they posed. For instance, in the legal field, statistics are frequently used as evidence in trials.
However, these statistics are often presented by expert witnesses from other fields, like psychology or social sciences, or even by the lawyers themselves—not by statisticians. This has inevitably led to erroneous or, at the very least, incomplete presentations of statistical evidence in many U.S. court cases.
Another example is an interview with Robert Keller, the acting Director of the U.S. Census Bureau, during the 2023 Joint Statistical Meetings (JSM) convention in Ontario, Canada. Keller was asked what he could do to improve communication between the lower levels of the Bureau and its leadership, particularly regarding data collection concerns. The questioner, a subcontractor during the 2010 Census, recounted his experience: while working to locate uncounted individuals, he questioned what would happen if one of the laptops used in the process broke.
After raising his concerns, he was told, “We don’t tell the agency their plan is about to fail,” and his findings were suppressed. The question posed to Keller was how to create a more welcoming environment for those down the chain to voice concerns.
What I’m trying to convey is that as a data analyst, working on independent projects will be vastly different from working within an organization, where your experience will depend on its capabilities and goals. The variety of goals in data analytics is so broad that it’s impossible to master all statistical methodologies.
Depending on the organization, you might need to learn coding in C++, JavaScript, PowerBI, or Tableau. To students of statistics: Your responsibility isn’t to learn everything but to grasp just enough of a set of topics to become productive. Be prepared to learn new programming languages or methodologies as needed.
You may never be fully equipped to understand the whole story with statistics, but you will always be capable of discovering something new by focusing at the task at hand. And that’s what the practice of statistics is ultimately about and what makes it so interesting. It requires creativity and resourcefulness. There is no perfect analysis and the best surveys you take are after you analyze the results of your previous survey. So just collect data and see where it takes you.
2. Find mentors
For the average college student, there is probably a small percentage of them who would say they have ‘a mentor’. But these people do not necessarily have to be people you know personally, just people who add perspective to how you should approach data analysis. Additionally, the mentors you should pick are ones that align with your interests, or at the very least, they can help you pursue your interests. For example if you want to be a good writer of statistics, you should probably be a good reader of statistics. If you want to be a good communicator of statistics, find people who can communicate statistics well, and mimic them.
A few names for me that come to mind are:
- Andrew Gelman - Colombia University (StatModelling Blog)
- David J. Hand - Imperial College London (Dark Data, The Improbability Principle)
- Nate Silver - Statistician and Political Analyst (The Signal and the Noise: The Art and Science of Prediction)
- Peter L. Bernstein - Historian and Economist (Against the Gods: The Remarkable Story of Risk)
The most important thing is learning from those who have more experience than you. Although the world is available online - it takes skills and experiences to determine what information is useful and what is not.
To most people, mentors are going to be the professors that you encounter during your educational years. This is the time to ask questions. These people have made mistakes, they have gone through love and loss, altogether they have cycled through many different jobs.
Most professors will be willing to answer any of your questions and then some after you take the steps to develop anything more than the typical superficial relationship they have their students. Showing them that you care about them and appreciate the efforts they put in a teachers go a long way. To me, showing kindness and respect has led to wonderful recommendation letters, and even recommondations to internships and jobs.